A multimodal dataset for network epidemiology from a two-week long outbreak simulation at Wenzhou-Kean University
By Andrés Colubri —
Together with faculty and students from Wenzhou-Kean University (WKU) in China, we recently organized and ran the largest and longest Operation Outbreak (OO) research simulation, involving nearly 800 students from November 20 to December 4, 2023. This real-world experiment offered a unique opportunity for WKU students to contribute to infectious disease research by making daily decisions that could affect the progression of the simulated outbreak, while generating a valuable multimodal dataset for subsequent analysis. This dataset includes anonymous contact traces between participants, full transmission chains of the virtual pathogen, participants’ choices, and even synthetic genome sequences of the pathogen. In this post, we will delve into the details of this WKU simulation and provide a brief overview of the dataset and ongoing analyses applying methods from network epidemiology.
Update: Since I wrote this article, the team in my lab carried out epidemiological modeling of the data from this simulation, and all resources (pre-print and code) are available through this repository.
Background and planning
This simulation was possible thanks to the support of Prof. Dale King and Dean Aloysius Wong at WKU. Planning started in the early Fall semester of 2023, when Prof. King was able to recruit a group of WKU students who were interested in participating in the project as simulation organizers and facilitators. Our initial goals were the following: reaching around 1,000 students, running the simulation during two complete weeks, and piloting new features to allow behavioral research with OO, inspired by discussions with our collaborators at the Vermont Complex Systems Center, Profs. Laurent Hébert-Dufresne, Jean-Gabriel Young, and Sarah Nowak, earlier that year.

The aim of having around 1,000 students engaged with the simulation for two weeks, while they were attending their normal classes and other school activities, represented a challenge. First, we realized that we needed to carry out a strong campus information campaign to get students interested as well as incentives for them to join the simulation and participate until the end of the two weeks. This was not a new challenge; when we run similar large-scale OO simulations at the campuses of Colorado Mesa University (CMU) in 2020 and Brigham-Young University (BYU) in 2021 (work that has been described in this paper), we also worked with local teams of students to ensure participation and engagement. The main difference with those prior experiences is that this simulation would involve more students and run for a longer period. Furthermore, there have been significant changes in the technology behind OO since 2021. For instance, that year we adopted the open-source Herald proximity library to enable contact detection over Bluetooth, and ported the mobile app to the Flutter framework for easier development and maintenance. We already tested these changes in the simulation at Walter Johnson High School in October 2022, which we wrote about in an earlier post. This was the largest OO simulation to date at the time, with 400 students, but it also was an immersive educational simulation for middle and high schoolers that went on only for a few hours. So, at WKU we really put the system to test by running the app and underlying infrastructure for 14 days non-stop.

Second, we wanted to try out some ideas that we were discussing with our colleagues from the University of Vermont, who are experts in network epidemiology and health policy, around how OO could provide a platform for evaluating non-pharmaceutical interventions, such as contact tracing and quarantine, and the potential correlation between health-seeking behaviors and network structure. This could be done through “experimental games” that may help understand behaviors during an infectious disease outbreak. We considered how to gamify health-related decision-making in the OO app, in such a way that students would need to make choices in the simulation on a regular basis, mirroring conflict between individual and group benefits, just like in a real pandemic. Based on these considerations, we eventually implemented a point-based system where students could collect points by deciding to “quarantine” or not at the beginning of each day, and then use those points to purchase (virtual) protective items and “medication”. (Of course, it would have not been reasonable to ask students to quarantine by physically restricting their movement, instead they “quarantined” by selecting a button in the app, which made their avatars invisible to nearby participants.) The goal with the point system was to provide a quick mechanism for students to get points without distracting them from their daily school activities, but still offering a sense of personal investment in the simulation, as their points informed a school-wide leader board that was used to award prizes to the top-scoring students once the simulation ended. In this way, the app involved a significant number of new features that we had developed very quickly in time for the starting date of November 20th.
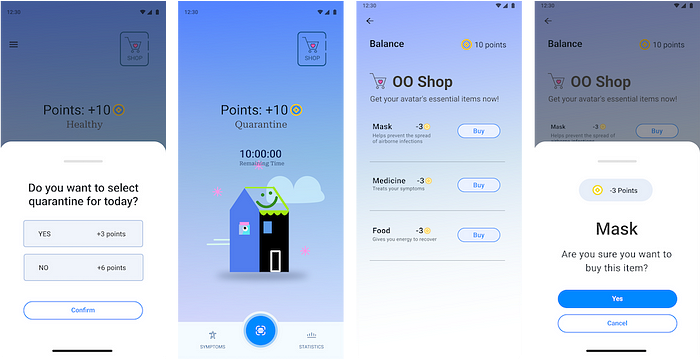
Promoting the simulation at WKU
Current enrollment at WKU is around 4,000 students, so getting a quarter of those to join the simulation prompted us to design a multi-faceted strategy to reach this target. We created several informative materials, including a project website, WeChat groups, and digital and physical fliers. The physical fliers were posted at locations throughout the campus with high student circulation, and the local organization team also setup an information desk where interested students could stop by to learn more about the simulation.
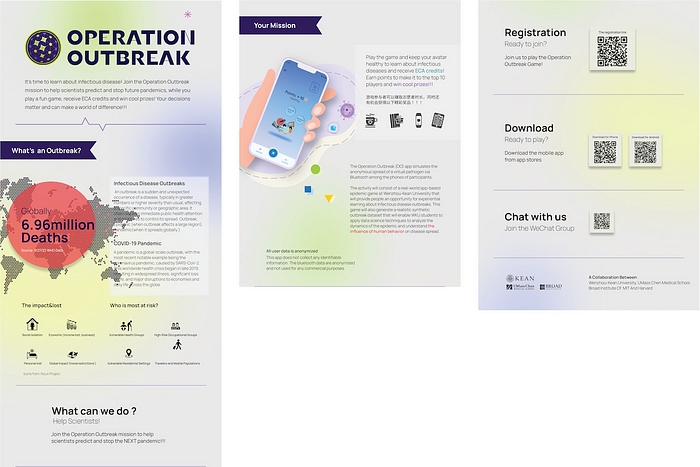
We created a registration form for interested students to enter their name, school email and ID, with this data handled exclusively by the local organization team. They would regularly report the total number of registered students to us, so we could get a sense of how many students were considering participating in the simulation. Most students also joined the WeChat group, which made it quite easy for them to ask questions about the simulation.
In addition to providing all these information channels, we implemented several rewards for participating students. First, the school agreed to offer extra-curricular credits to any student who participated in the simulation; this represented a tangible benefit with academic value, so we think it made a big difference. Then, the point system in the app enabled us to build a live leader board that students could check from their app. Those making into the top ten at the end of the simulation would get prizes, ranging from an electric scooter to gift cards. We believe that there was a sufficiently strong incentive for students to try to get a high score, however, this would come into conflict with the situation posed by the simulated outbreak in two ways: one, individuals trying to maximize their score by skipping daily quarantine (since quarantining resulted in fewer points) or not using points to purchase protective items (i.e.: masks), would increase their chance of exposure and, as result, could lose a major fraction of their collected points. Two, and more indirectly, if the disease spreads more widely due to fewer students adopting protective behaviors such as quarantine or masking, then the risk of exposure would increase for all. Finally, we also implemented a raffle mechanism in the app that would randomly pick several students each day (between 4 and 8), who could claim a ticket for a free latte at the campus coffee shop. This provided an additional incentive for everybody, irrespective of whether they were doing well with their score or got infected — simply by keeping using the OO app throughout the entire simulation.
Right before the simulation started, we posted a summary of the instructions in both Chinese and English, as well as a poster detailing the rewards that students could win during the simulation (daily raffle and final prizes for the top-scoring students).

The OO-WKU simulation — during and after
By the time the simulation was set to start, we had slightly over 1,000 registered students, with 794 joining over the next few days, which is a good retention rate for this kind of voluntary school activities. In general, the simulation went according to the plan, with a few technical glitches affecting a small fraction of users (we will go over these in more detail at the end of the post). We developed a couple of custom web tools specifically for this simulation, one to run the daily raffles and post the results on a website as well as sending a notification through the app, and the other to display the leader board. This leader board was available online and through the app.
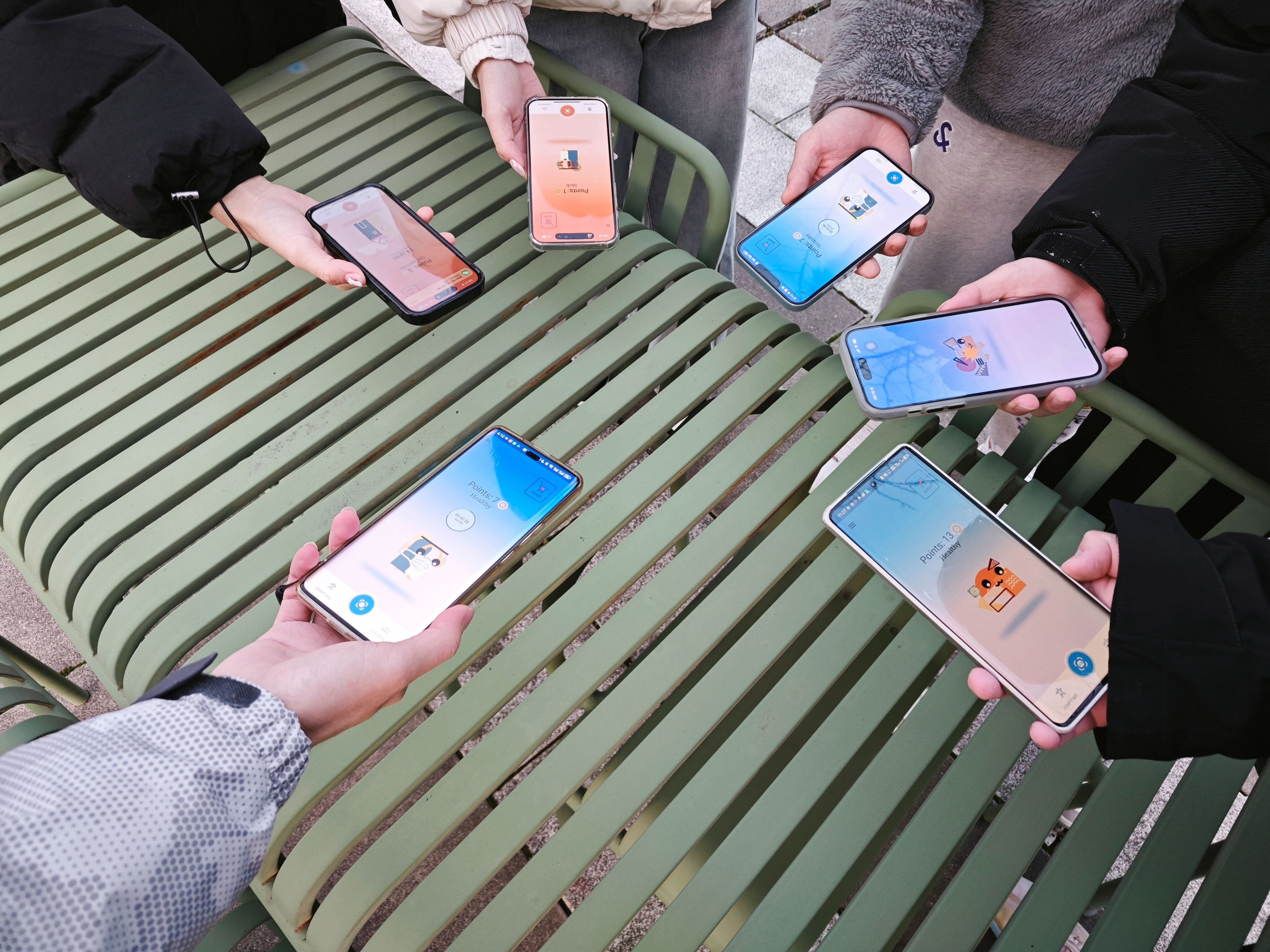
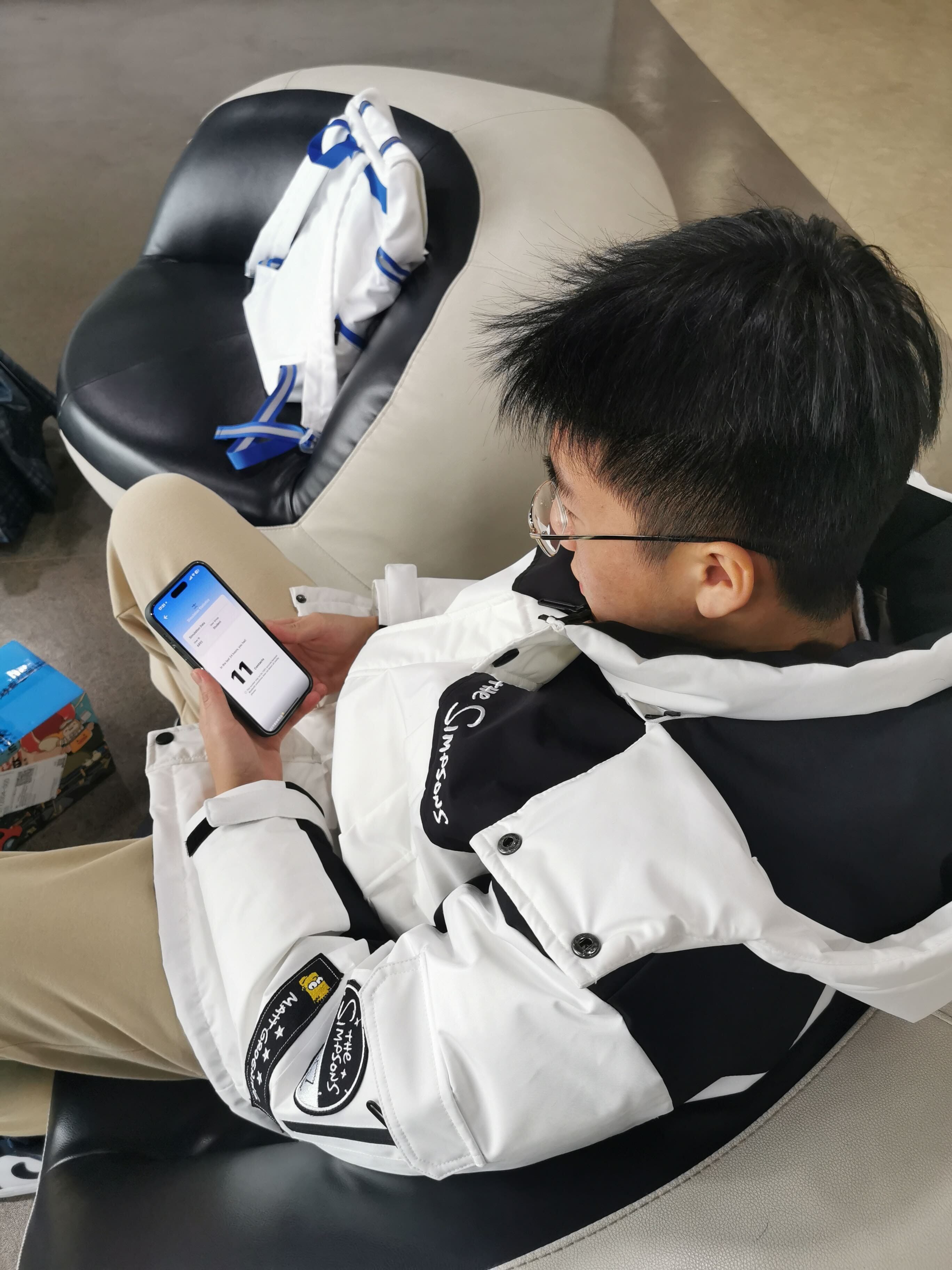


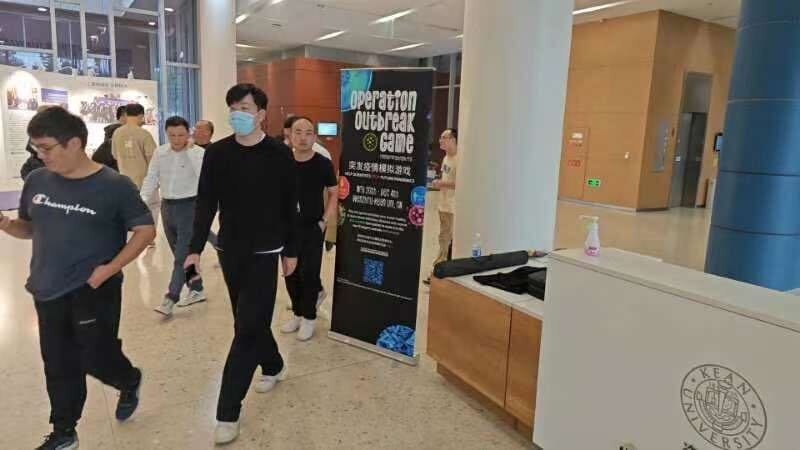
A week after the simulation had ended, we had the chance to talk about the activity and show some early results during an online seminar. A few days later, Prof. King handed out the prizes to the top-scoring students.
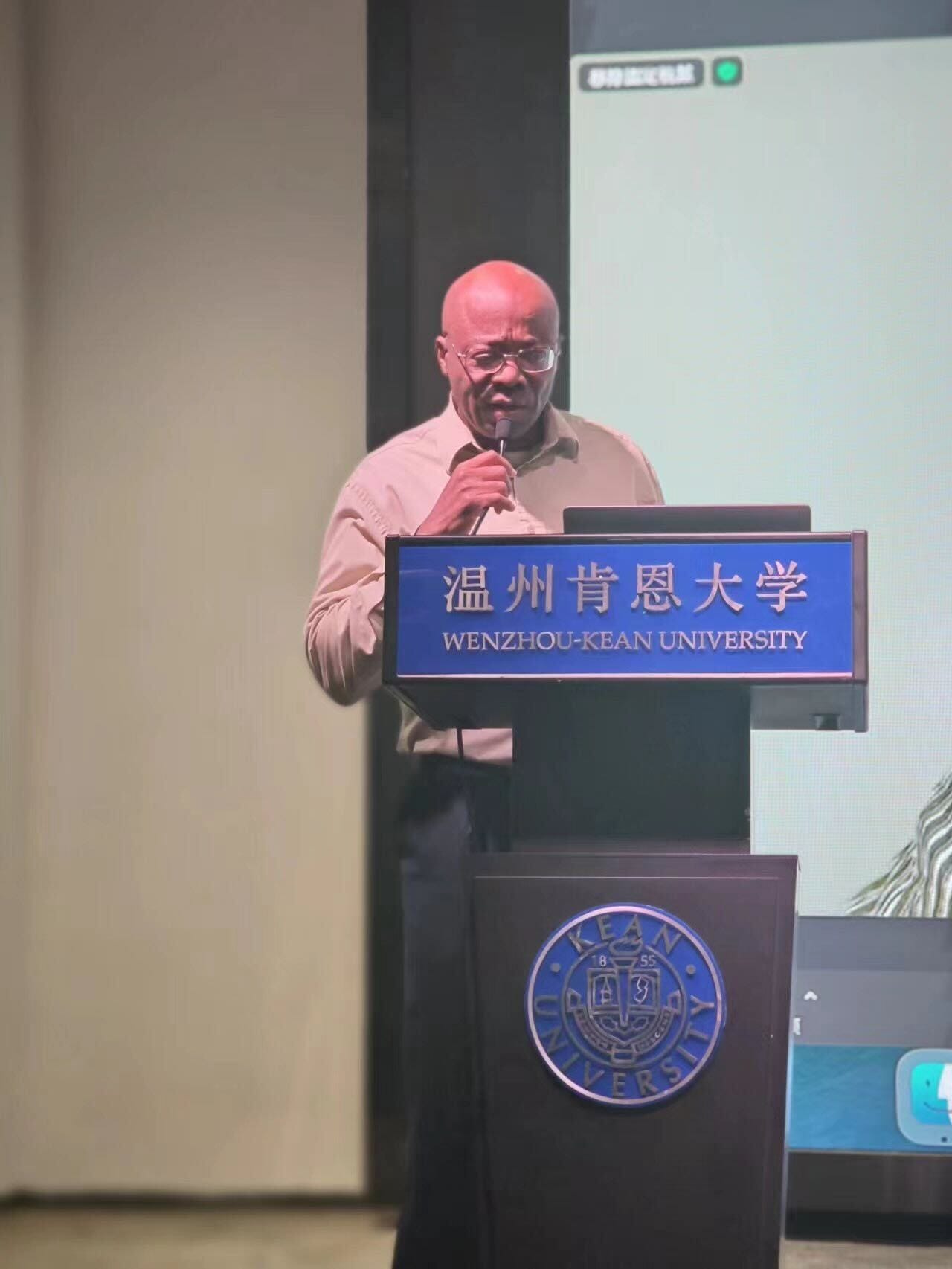

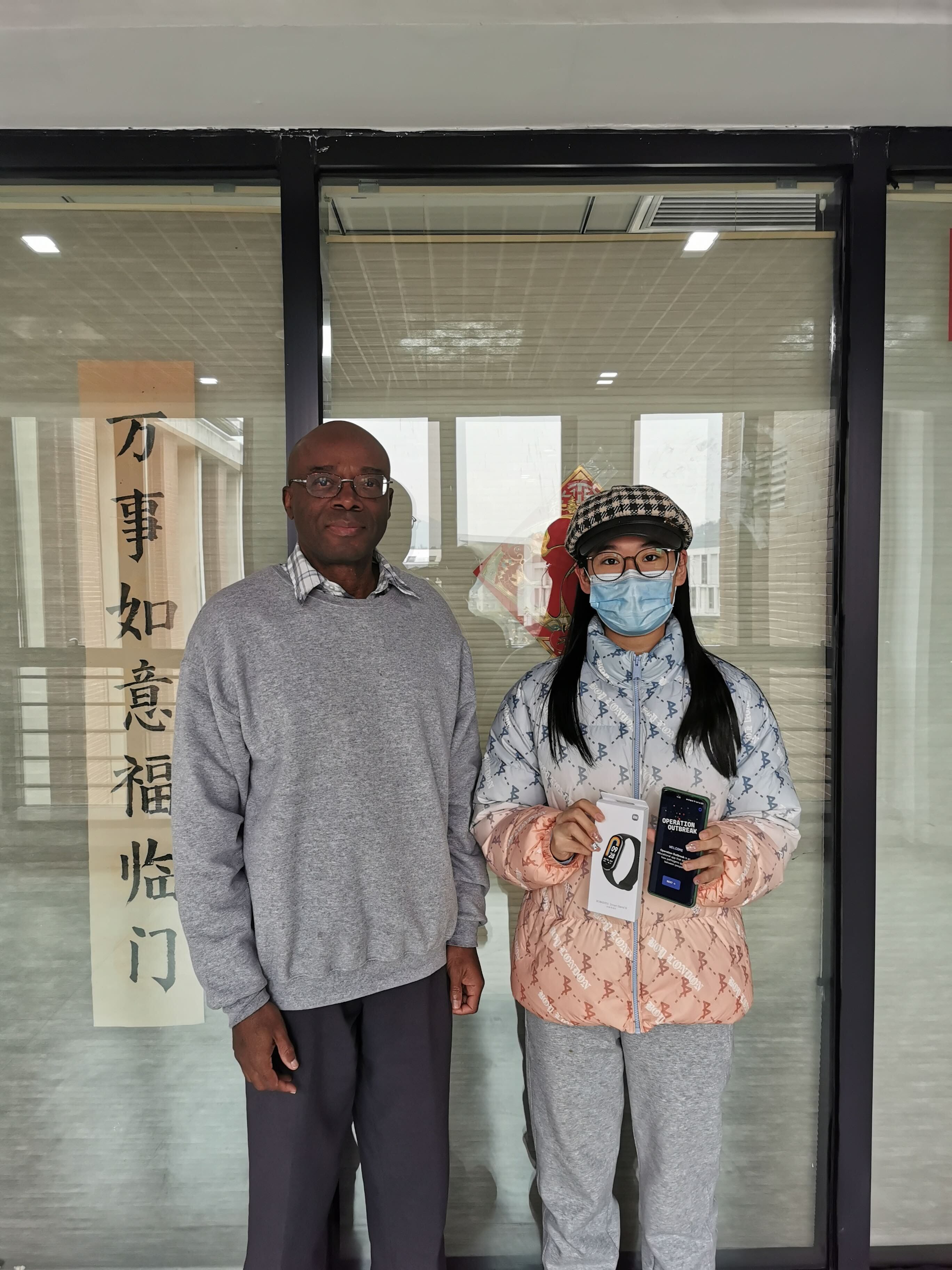
A preliminary look at the data
The raw data from the OO-WKU simulation included contact traces for 794 participating students over 14 days, simulations events such as infections (and re-infections), individual quarantining, and disease outcomes (“recovery” and “death”). This generated a total of 2,020,163 individual entries in the database. The app also mutated a short DNA fragment 213 nucleotides long every time one person infected another starting from an “ancestral” sequence that was used to seed the index cases. Even though this sequence data did not have any impact on this simulation, we still generated the phylogenetic tree from all the cases.
Anybody can visualize the contact data very easily with an interactive online outbreak viewer (OV) created by Fathom Information Design, which can be accessed from the browser by clicking here:
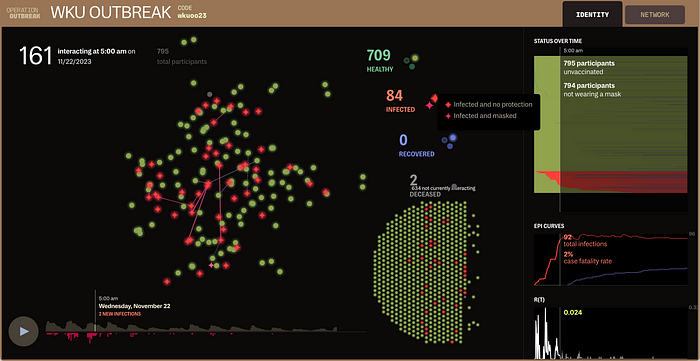
This viewer has a few different modes to show the data, the default in the identity mode where all the contacts are displayed as they happen over time, with a few additional charts that represent the entire data. The network and individual models can be accessed from the main page to examine the overall transmission network and the contact layers of individual participants.
Another novel data modality in this dataset consists of health-related perceptions before the simulation and actions taken by the students during the simulation. More specifically, students were asked to complete a survey immediately after joining the simulation, where they had to enter their user ID (a randomly-generated 4 digits ID in the app) and respond questions about perceptions regarding benefits and costs of quarantine, adapted from a survey described in this paper published in 2009 following the H1N1 pandemic. Over the course of the two weeks of the simulation, we recorded the daily count of students who decided to quarantine and buy masks and medicines each day (the medicine item would make a severely ill player to become less sick, this in turn reducing the probability of a fatal outcome).
Network epidemiology analysis of the WKU data
Network epidemiology combines the biology and statistics of epidemiology with the theory of networks to improve our understanding of how infectious diseases spread in a population. It captures human behavior by measuring the networks of connections between individuals, and this is precisely the kind of data that OO can generate during the simulated outbreaks. Our ongoing analyses of the WKU dataset (and results from the earlier experiments at CMU and BYU) suggests that OO simulations are able not only of capturing aspects of human behavior that are an explicit component of the simulation mechanics (e.g.: players deciding to quarantine or use masks) but also of “encoding” human behavior into the structure of the network. We will be writing a manuscript reporting these results in detail and adding the link here once it is available; for the time being, we will give a very brief overview of our preliminary results below.
We start by constructing the contact network for the entire WKU simulation. We do this by adding up the total time each pair of students were in contact according to the app, and then using a node to represent each a student (we removed nodes with no contacts) and connecting every pair of nodes for students who were in contact with an edge whose line width reflects total contact duration. This diagram of this network is shown in the following figure:

In this diagram, we can see that a few nodes have a high number of contacts, while many other nodes have far fewer contacts. As it turns out, because the distribution of the number of contacts per node follows a power law (the probability of having many contacts decreases exponentially), the contact network from the OO simulation at WKU is “scale-free”. This is very important because scale-free networks, unlike other kinds of networks, don’t have a critical level of interaction required for an outbreak to take place. This means that, even with minimal contact between individuals, an infectious pathogen will spread through the network. This is consistent with earlier studies of contact networks measured with mobile devices, such as in the FluPhone project, where for shorter inter-contact times (less than 12 hours), the distribution of contact times follows a power law.
The next video shows how the virtual pathogen spread through the WKU network during the simulation. In this video, a blue node is healthy, while orange means infected and green, recovered:
After the initial index cases (randomly seeded by the app) and a few transmissions, there was a large increase in cases once the pathogen reached the highly connected nodes in the center of the network, likely indicating one or more “super spreading” events. During these events, individuals called super spreaders cause many more new infections than the rest of people. This also makes sense given other properties we were able to identify in the WKU network so far. One of them being that nodes that were alike (with regards to preferences about quarantine or number of contacts) tended to be connected between each other (which means the network is “assortative”, using the language of network science). The other property is that the super spreading events in the WKU simulation follow similar patterns to those occurring in real-world outbreaks. We applied the methods to study super spread epidemiology described in this recent paper that looked into 382 transmission trees for 16 directly transmitted diseases, including COVID-19, and the transmission tree at WKU has a similar proportion of super spreaders with comparable pathogens (we did set the parameters of the WKU pathogen to be similar to those of the Omicron variant of the SARS-CoV-2). This tree is shown in the figure below:
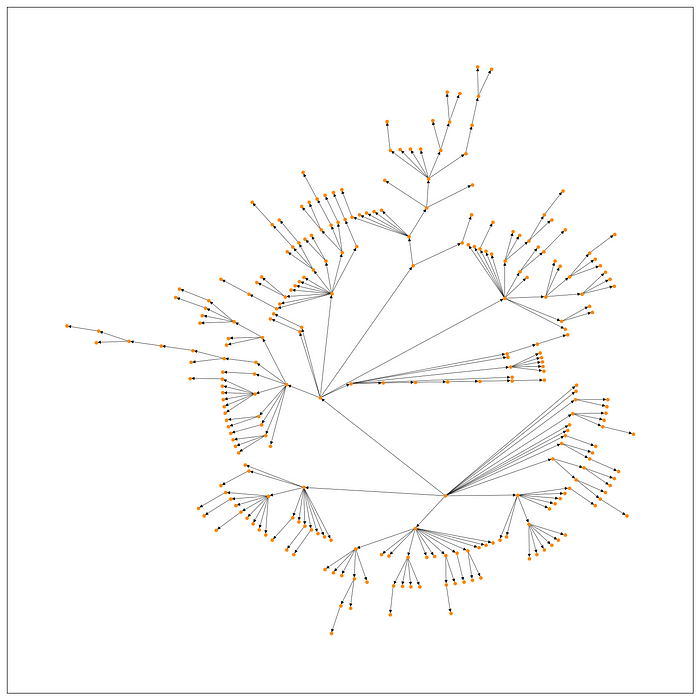
Conclusions
The OO simulation at WKU was a unique opportunity to pilot the platform at scale, both in number of participants and duration of the simulation. With nearly 800 students participating for two weeks, the simulation was not only one of the largest but also one of the most comprehensive to date, incorporating behavioral experiments and generating valuable multimodal data. This simulation exemplified the potential for interdisciplinary collaboration and offered a novel opportunity for students to gain practical experience in epidemiological data generation and analysis.
In addition to testing the OO platform and showing that is capable to run for extended periods of time and generate multimodal datasets for network epidemiology analysis, we also learning that the dataset is highly realistic in terms of the outbreak dynamics and recapitulates patterns that were observed in real outbreaks. The analysis is ongoing, and we anticipate arriving at additional findings. Furthermore, several simulations are in the planning stage, which will both refine what we did at WKU and add additional data streams. By pushing the boundaries of what is possible, we continue to advance our understanding of disease dynamics and improve our preparedness for future health crises. For more information on Operation Outbreak and its impact, visit their website at https://operationoutbreak.org/.
Acknowledgments
We would like to thank WKU students and faculty who made this simulation possible, members of the Colubri Lab who worked on the implementation of all the features used in this simulation, the colleagues at UVM who are collaborating with us on the data analysis, the developers of the Herald library, Fathom Information Design for their work on the visualization tools, and all members of the OO team at the Broad Institute for their support.